Abstract
Learning probability distribution is an essential framework in classical learning theory. As a counterpart, quantum state learning has spurred the exploration of quantum machine learning theory. However, as dimensionality increases, learning a high-dimensional unknown quantum state via conventional quantum neural network approaches remains challenging due to trainability issues. In this work, we devise the quantum sequential scattering model (QSSM), inspired by the classical diffusion model, to overcome this scalability issue. Training of our model could effectively circumvent the vanishing gradient problem to a large class of high-dimensional target states possessing polynomial-scaled Schmidt ranks. Theoretical analysis and numerical experiments provide evidence for our model’s effectiveness in learning both physical and algorithmic meaningful quantum states and show an out-performance beating the conventional approaches in training speed and learning accuracy. Our work has indicated that an increasing entanglement, a property of quantum states, in the target states, necessitates a larger scaled model, which could reduce our model’s learning performance and efficiency.
Publication
Physical Review A
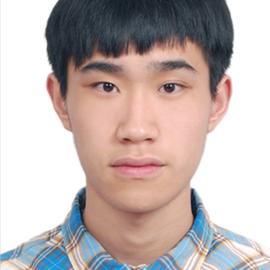
PhD Student (2023)
I obtained my BS and MS degrees in physics from the University of Melbourne. My research interests include distributed quantum computing, quantum entanglement and quantum machine learning.
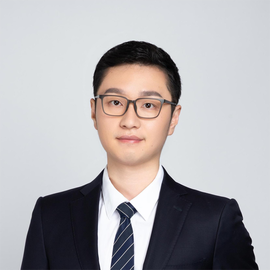
Associate Professor
Prof. Xin Wang founded the QuAIR lab at HKUST(Guangzhou) in June 2023. His research primarily focuses on better understanding the limits of information processing with quantum systems and the power of quantum artificial intelligence. Prior to establishing the QuAIR lab, Prof. Wang was a Staff Researcher at the Institute for Quantum Computing at Baidu Research, where he concentrated on quantum computing research and the development of the Baidu Quantum Platform. Notably, he spearheaded the development of Paddle Quantum, a Python library designed for quantum machine learning. From 2018 to 2019, Prof. Wang held the position of Hartree Postdoctoral Fellow at the Joint Center for Quantum Information and Computer Science (QuICS) at the University of Maryland, College Park. He earned his doctorate in quantum information from the University of Technology Sydney in 2018, under the guidance of Prof. Runyao Duan and Prof. Andreas Winter. In 2014, Prof. Wang obtained his B.S. in mathematics (with Wu Yuzhang Honor) from Sichuan University.