Abstract
Accurately estimating high-order moments of quantum states is an elementary precondition for many crucial tasks in quantum computing, such as entanglement spectroscopy, entropy estimation, spectrum estimation, and predicting nonlinear features from quantum states. But in reality, inevitable quantum noise prevents us from accessing the desired value. In this paper, we address this issue by systematically analyzing the feasibility and efficiency of extracting high-order moments from noisy states. We first show that there exists a quantum protocol capable of accomplishing this task if and only if the underlying noise channel is invertible. We then establish a method for deriving protocols that attain optimal sample complexity using quantum operations and classical postprocessing only. Our protocols, in contrast to conventional ones, incur lower overheads and avoid sampling different quantum operations due to a novel technique called the “observable shift method”, making the protocols strong candidates for practical use on current quantum devices. The proposed method also indicates the power of entangled protocols in retrieving high-order information, whereas in the existing methods, entanglement does not help. We further construct the protocol for large quantum systems to retrieve the depolarizing channels, making the proposed method scalable. Our work contributes to a deeper understanding of how quantum noise could affect high-order information extraction and provides guidance on how to tackle it.
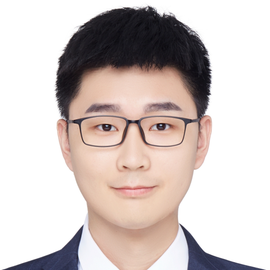
Research Assistant
I obtained my MS degree in Physics from Imperial College London. I was an intern at Baidu Research under the supervision of Prof. Xin Wang. I am currently a PhD student in quantum information at Osaka University. My research interests include quantum error mitigation, quantum information theory and quantum computation.
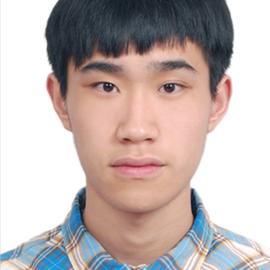
PhD Student (2023)
I obtained my BS and MS degrees in physics from the University of Melbourne. My research interests include distributed quantum computing, quantum entanglement and quantum machine learning.
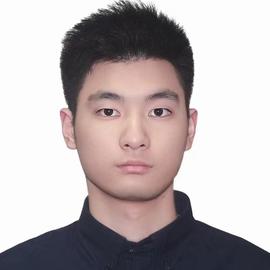
PhD Student (2023)
I obtained my BMath in AMath, CO & joint PMath from the University of Waterloo. My research interests include quantum information theory and quantum machine learning.
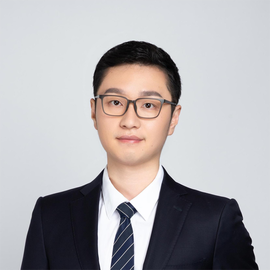
Associate Professor
Prof. Xin Wang founded the QuAIR lab at HKUST(Guangzhou) in June 2023. His research primarily focuses on better understanding the limits of information processing with quantum systems and the power of quantum artificial intelligence. Prior to establishing the QuAIR lab, Prof. Wang was a Staff Researcher at the Institute for Quantum Computing at Baidu Research, where he concentrated on quantum computing research and the development of the Baidu Quantum Platform. Notably, he spearheaded the development of Paddle Quantum, a Python library designed for quantum machine learning. From 2018 to 2019, Prof. Wang held the position of Hartree Postdoctoral Fellow at the Joint Center for Quantum Information and Computer Science (QuICS) at the University of Maryland, College Park. He earned his doctorate in quantum information from the University of Technology Sydney in 2018, under the guidance of Prof. Runyao Duan and Prof. Andreas Winter. In 2014, Prof. Wang obtained his B.S. in mathematics (with Wu Yuzhang Honor) from Sichuan University.